AI trick for cleaning up machine data
Solution for faulty training data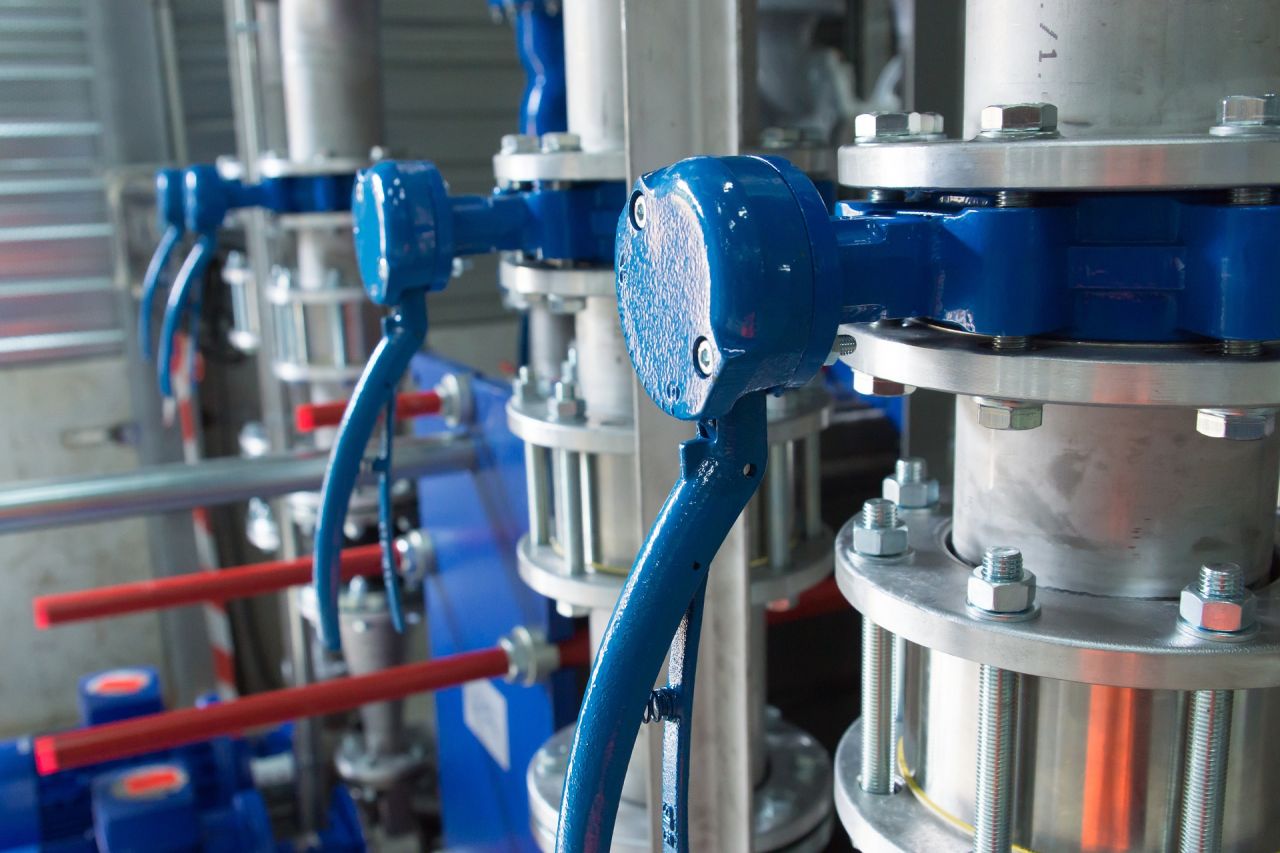
Researchers at the ZHAW School of Engineering have developed a method to detect anomalies and defects in machines more efficiently, even when AI training data is contaminated.
Detecting wear, defects and faults at an early stage: More and more industrial companies are using AI for these tasks. The principle applied here is called “learning from normality”. Algorithms are trained using data from perfectly functioning machines in order to detect deviations later on. In practice, however, there is often no completely error-free data available. As a result, the models can no longer distinguish between normal and faulty operating conditions.
To solve this problem, researchers at the ZHAW School of Engineering have developed a novel framework. It is based on a central observation: faulty data has a stronger influence on the performance of an AI model than normal samples. According to this principle, each data sample is assigned a score that measures its influence on the training. Samples with a high score are identified as potentially erroneous and removed from the training data. In tests, the data refined in this way achieved a comparable performance to manually cleaned data sets.
The ZHAW tested the method on a variety of machine types, including pumps, valves, fans and engines, and achieved promising results. In most cases, the framework was able to fully compensate for the lack of error-free training data.